Facility managers and buildings owners can use machine learning to control and, eventually, predict energy management use. That can save money on one of the largest financial factors of owning and managing a building.
Akshai Rao, vice president at Yardi, used his presentation at the 2019 BOMA International Conference & Expo, “Exploring Artificial Intelligence and Machine Learning: New Frontiers in Energy Management,” to demystify the technology, what it means and how it can be applied.
Artificial intelligence, he explains, is any technique that enables a computer to mimic human intelligence to learn from experience. A subset of that, machine learning includes statistical techniques to enable a computer to perform a task more efficiently as more data is received.
Going one subset further, deep learning is good for energy management, and is based on neural networks capable of learning from data sets that may be structured or unstructured.
Rao explains a few ways that artificial intelligence impacts commercial real estate:
- Building optimization: Dramatically reduce energy intensity and operational costs while improving tenant comfort
- Lead to lease: Nurture leads more effectively across channels while reducing expenses
- Customer service: Decrease response time across channels and increase accuracy and consistency of answers
There’s lot of opportunities in energy management of buildings to use artificial intelligence and machine learning to save money, create revenue in the form of rebates or prevent brownouts, Rao explains.
Although that’s in the future, you can implement incremental improvements in your building now by looking at the data you have and evaluate how you can save money, and use software and platforms sitting on Building Management Systems where you are generally in control.
“The goal of these platforms and software for buildings is to help you and your peers do your jobs on a more scalable fashion,” Rao says. “We need to view all these algorithms and technologies in that manner.”
[Read also: Connected Buildings Increase as Companies Prioritize Advantages]
To look at energy management, the manager needs to consider all the variables that happen in a specific time period for a building and make appropriate decisions in terms of how the energy is consumed.
Rao gives the example of a building where hundreds of people gather in the main lobby every Tuesday at 9 a.m. With machine learning, the model starts to learn that this happens every week and prepares accordingly. Systems can decide before 9 a.m. to appropriately cool the space rather than take corrective measures after 9 when the temperature has elevated from the people gathering.
Machine learning “effectively looks at when should we be aware of load increases on a building and how should we act and be proactive ahead of these load increases,” Rao says.
More Predictive Energy Management Solutions
Energy management solutions are the “perfect” use case for machine learning, Rao points out. Right now, machine learning in energy management is about looking at the inputs at very specific points in time and zones of a building.
If, for example, the ambient temperature is increasing, it assumes the load is increasing too and more people are coming into the area. To accommodate, systems react in specific and incremental ways to respond quickly and efficiently.
In the next few years, Rao suggests that machine learning can evolve to make more proactive and predictive decisions. The machine learning model could take information other information to determine how many people are in the building and where they might be. Examples of places it could pull information include:
- The example from the lobby every Tuesday morning
- Working with a scheduling system to see what meeting rooms will be in use
- Getting data from a parking gate about how many people are parked and might be in the building at the time
Determine Energy Management Savings
By not using machine learning for energy management solutions, building owners aren’t being as cost-effective as possible, Rao says. “By not managing more effectively, they are leaving a very large opportunity on the table. The owners [using machine learning] will be ahead and have excess returns,” he says.
[Related: Predict and Prevent Issues With Artificial Intelligence]
Coupled with uncertainty of utility rates over the next 10 years in some areas, machine learning should be a consideration if not already in use.
Rao suggests that to start seeing savings, building owners follow these three steps:
1. Take a portfolio-level view of properties.
Every building is different and will need its own solution, so look at everything to see where the most opportunity exists.
2. Benchmark internally and externally.
“The only way you will know what buildings need it the most is through benchmarking,” Rao says. He suggests benchmarking internally and using an external benchmarking tool like ENERGY STAR to get an apples-to-apples comparison across the industry.
3. Take an incremental approach to energy management improvement.
Once it’s determined what buildings or areas can benefit the most, bring in machine learning to reduce energy consumption where the return makes the most sense, Rao advises.
BUILDINGS Podcast
Rebates, Tax Incentives and Financing for Building Upgrades
[podcast]
Looking for tips on how to finance your building upgrades with rebates and tax incentives?
Janelle Penny speaks with Emily Naden, director of federal affairs, and John Bryant, vice president of advocacy and building codes, both from BOMA International, about where you should start as well as more of what you need to know. Listen now >>
Human Help With Machine Learning
While machine learning can help building owners and property and facilities managers with energy management savings, technology can only take you so far.
“Because the platforms are algorithm-driven, it’s managed by humans,” Rao says. “The goal is to allow the platforms and algorithms to do the best type of situations and scenarios and allow the humans to apply the intelligence where there are acceptations.”
Incorporating machine learning in the energy management process can free property and facilities managers to focus on other parts of the building or manage more buildings. No matter what managers are doing with their time, Rao emphasizes that for energy management, human intelligence and context won’t go away.
“There is nothing that knows the building better than the chief engineer and property manager,” he says.
More BOMA 2019 Coverage:
About the Author
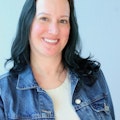
Valerie Dennis Craven
Content strategist and writer
Valerie Dennis Craven is an experienced writer of commercial and residential buildings and interiors, having previously served as Editorial Director for both BUILDINGS and i+s. Valerie enjoys writing about technology and how it impacts users in the built environment.