The Real Value of Digital Twins in Smart Buildings
Key Takeaways:
-
Digital Twins Deliver Real Value: 62% of organizations report “immense value” from digital twin technology, according to Hexagon’s industry report.
-
Predictive Maintenance Prevents Major Failures: AI-powered digital twins monitor equipment like HVAC and elevators, identifying anomalies before costly breakdowns occur.
-
Energy Modeling Cuts Waste in Mixed-Use Buildings: Smart simulations help reduce energy consumption by optimizing lighting and HVAC usage based on occupancy and weather data.
-
Deployment Challenges Exist: Legacy systems, sensor calibration issues, and limited access to skilled professionals can complicate implementation—but can be overcome with planning.
For many smart building owners, a digital twin is not just a buzzword but a proven technology that has demonstrated tremendous value in the short time it has been available. The creation of virtual replicas of physical assets, fueled by real-time data and artificial intelligence to perform advanced analytics, is becoming increasingly sophisticated and accurate. In fact, a recent Digital Twin Industry Report from Hexagon shows that 62% of respondents claim “immense value” from their digital twin initiatives.
How are these companies deriving such value? Let’s examine a couple of relatable smart building use cases illustrating how digital twins enhance efficiency through predictive maintenance and energy modeling within typical smart buildings.
Use Case 1: Predictive Maintenance in a Class-A Office Building
Our first use case example is a Class-A office tower, where building operators implement a digital twin to monitor critical systems like heating, air conditioning components, and elevators. With the help of a network of IoT sensors, the digital twin collects real-time data on equipment performance, looking for changes in baseline behavior, including aspects such as motor vibrations, temperature fluctuations, and humidity spikes.
AI analytics is used to predict potential failures, anticipate when failures will occur, and offer guidance on precisely what maintenance needs to be performed. This type of proactive and intelligent modeling has the potential to eliminate major outages and to identify and remediate small mechanical faults at a lower cost compared to waiting until these small issues turn into much larger (and costlier) incidents.
Use Case 2: Energy Modeling in a Mixed-Use Retail Complex
A second relatable use case involves a mixed-use retail complex that includes retail shops, offices, and restaurants. Here, building operators deploy a digital twin to optimize energy consumption across the diverse tenants. The digital twin aggregates data from smart meters and occupancy sensors and collects real-time weather forecast data from the internet to model energy usage across smart lighting, HVAC, and escalator management platforms. By simulating hundreds of different scenarios within the digital twin, operators learn that adjusting HVAC settings during low-occupancy evening hours significantly reduces energy waste, aiding compliance with local and state regulations and lowering operating costs.
Digital Twin Challenges and Considerations
While digital twins deliver clear benefits, it must be pointed out that smart building owners, operators, and integrators face adoption challenges. For predictive maintenance use case examples, integrating IoT sensors with legacy systems can end up being a complex process, often requiring more time than anticipated, along with significant investments that likely were not initially accounted for. Additionally, poor data quality, such as inaccurate or mis-calibrated sensors, can unintentionally skew AI predictions, leading to tedious troubleshooting and manual adjustments.
As for energy modeling use cases, challenges can occur when attempting to build baseline models that are accurate. Doing so requires high-quality historical data over a sustained period of time, which older buildings and IT/OT equipment may struggle with.
It’s also important to note that smart building digital twins are still a relatively new concept for building operators and integrators. Thus, a seamless digital twin deployment requires finding the right skillset. This can be a scarce resource depending on the market or geographical location.
That said, with the right planning, coordination and patience, these challenges can be overcome, giving building owners the ability to harness digital twins for smarter, more efficient operations.
Conclusion
Despite some remaining early-adoption hurdles to overcome, digital twins are transforming smart buildings, further driving cost, resource, and energy efficiencies through predictive maintenance and energy modeling. From preventing costly HVAC and elevator failures in our Class-A office building to cutting energy usage and costs in mixed-use retail complexes, these virtual replicas enable data-driven management. As long as integration issues, data quality, and strategic planning are conducted with trained professionals who have experience with digital twin technologies, significant smart building operations have significant potential.
Next Steps for Building Owners and Smart Tech Integrators:
-
Assess Infrastructure Readiness: Conduct a digital audit to evaluate existing IoT sensor networks and system integration capabilities.
-
Invest in Quality Data Collection: Prioritize high-quality, calibrated sensors and ensure consistent historical data capture for energy modeling accuracy.
-
Develop Predictive Maintenance Protocols: Partner with vendors or integrators who can help tailor AI-based analytics for your specific equipment.
-
Train or Hire Specialists: Identify talent with digital twin implementation experience or upskill internal teams to manage the technology.
-
Start with Pilot Projects: Test digital twin use cases (e.g., HVAC or energy optimization) on a smaller scale to validate ROI before expanding.
-
Plan for Ongoing Optimization: Treat digital twin deployment as an evolving strategy—continuously refine models based on performance feedback and operational insights.
About the Author
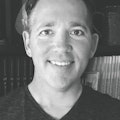
Andrew Froehlich
Contributor
As a highly regarded network architect and trusted IT consultant with worldwide contacts, Andrew Froehlich counts over two decades of experience and possesses multiple industry certifications in the field of enterprise networking. Andrew is the founder and president of Colorado-based West Gate Networks, which specializes in enterprise network architectures and data center build-outs. He’s also the founder of an enterprise IT research and analysis firm, InfraMomentum. As the author of two Cisco certification study guides published by Sybex, he is a regular contributor to multiple enterprise IT-related websites and trade journals with insights into rapidly changing developments in the IT industry.